How the World's Biggest Lenders Use Machine Learning
6/16/2021
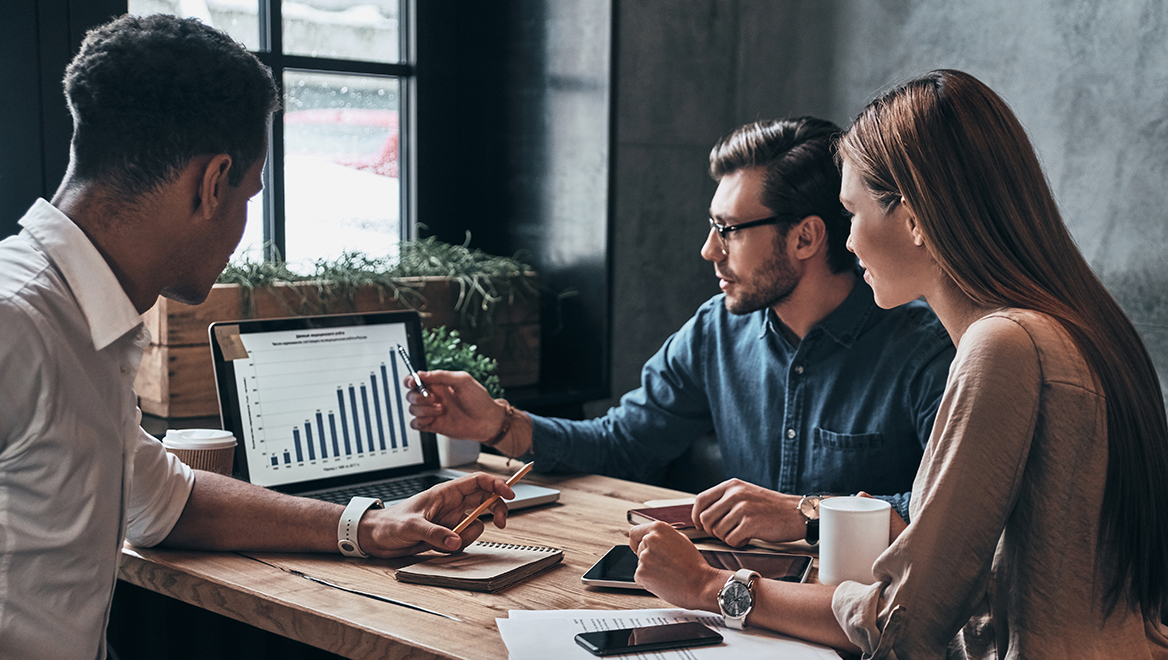
See machine learning in action and discover how lenders forecast creditworthiness and improve AML practices.
Financial institutions are finding success using machine learning in everything from chatbots to high-frequency trading techniques. Lenders also notice the benefits of artificial intelligence and machine learning-driven models.
LEARN MORE
Gain new information to effectively challenge machine learning models in credit risk management.
Contact RMA to find solutions that can help you and your institution.
Machine learning, defined as artificial intelligence used to construct mathematical models to make predictions[i], has the potential to generate value of more than $250 billion in the banking industry, according to the McKinsey Global Institute[ii].
These algorithms recognize patterns in large datasets and leverage those insights to determine what to do next while improving with each repetition of a task, according to the Institute for the Future (IFTF), Future of Connected Living – Augmented Humans in a Networked World[iii].
Among financial institutions, there is strong interest in leveraging machine learning to improve accuracy, lower costs, and increase efficiency by automating manual tasks.
The Benefits of Automating Credit Decisions
Among the world’s biggest lenders, Mitsubishi UFJ Financial (MUFG) began digital transformation initiatives relating to the use of machine learning and AI as of its fiscal year 2019 (ending March 31 of the following year). [iv] MUFG is one of the largest Japanese banks with total assets of $3.1 trillion. In the US, MUFG has its wholly owned subsidiary, Union Bank.
Notably, the Japanese financial group’s “on-line data lending” business model includes an AI-based credit scoring system. The scoring system is not based on financial data such as financial statements rather it evaluates corporate account activities, which can allow the bank to make faster lending decisions by relying on real-time data inputs and meeting urgent financing needs of corporate clients.
Similarly, MUFG launched a housing loan credit assessment service backed by AI credit assessment technology. The banking group disclosed that the AI credit assessment tool reduced loan officers’ data input burden by 50% with less time spent on each loan application for assessments. It also saw an increased number of loan applications from potential borrowers.
Mike Hepinstall, Partner at Oliver Wyman who leads the firm’s Financial Services Quantitative Analytics team in the US, says credit decisioning models using machine learning techniques can not only help lenders lower expected loss rates but also increase credit approval rates, speaking at Global Consumer and Small Business Risk Virtual Conference hosted by the Risk Management Association (RMA) in May 2021.
Improving AML Processes
Kevin D. Oden, Ph.D., Managing Director of RMA Model Validation Consortium, also notes that large banks are adopting the use of machine learning models to increase their operational efficiency.
Suspicious activity reports, or SARs, are an increasing burden on banks and other money services entities. SARs are reports about suspicious or potentially suspicious activity regarding money-laundering or fraud that financial institutions and those associated with their business, must file with the Financial Crimes Enforcement Network (FinCEN).
Oden indicates Bank Secrecy Act (BSA) and Anti-Money Laundering (AML) models have a high false-positive rate. A false positive is an alert that does not represent a possible case of financial crime. “This makes for a lot of manual interaction when institutions have to sift through the false positives to determine whether they are going to produce SARs,” he adds.
Conversely, a false negative is an instance of financial crime that has occurred without detection by the model. Financial institutions that fail to identify illicit activity and submit SARs may face penalties ranging from regulatory criticism and even sanctions and fines.
The International Finance Corporation’s Good Practice Note[v] states, if specific risks are managed properly, machine learning technology can be used to further refine processes for detecting patterns of suspicious transactions by learning from the experience of detecting true positives. The note adds machine learning technologies can identify complex patterns and nonlinear relationships and analyze unstructured data sources. When it is applied to transaction monitoring, it can detect suspicious activity more accurately.
A report by IBM[vi] also shows that by using machine learning techniques, a top 20 US bank decreased enhanced due diligence (EDD) investigation time by 60% or more. EDD processes occur when compliance officers gather additional information to verify the identity of a client to mitigate risks associated with the client. EDD is playing a key role in banks’ BSA and AML program because it is an extensive and ongoing effort to define customers affected by the risk profile and risk tolerance of the lenders.
The case study by IBM shows two challenges lenders face: first, they use a lengthy review process that is reliant on manual data entry. Second, due to the subjective nature of these investigations, the results are often inconsistent, which leads to a time-consuming audit process.
According to Oden, when institutions automate manual processes, it enables subject matter experts to focus on the most cogent problems. “That is where machine learning and technology can add value and reduce cost. People are thinking about this and starting to move forward rather quickly,” he notes.
The Risks
Oden says that the framework for machine learning model validation adopts many of the same practices for validating more traditional models. “When you think about things like cross-validation, dimension reduction and clustering, they are not new,” he adds.
Still, model risk arises with the use of machine learning techniques. In an interview with Fran Garritt, Director of Credit Global Markets Risk and Securities Lending at RMA, examining the challenges of model risk management with a focus on machine learning usage, Oden notes that the training data selection process for certain machine learning models may be subject to biases which may result in unfair outcomes. Oden expresses concern for modelers that may combine development methods without having a full understanding of their strengths and shortcomings.
Regulators in the US also notice the evolving landscape and the need for responsible development and use of machine learning. At the AI Academic Symposium on January 12, 2021, Federal Reserve Governor Lael Brainard [vii] stated, “As regulators, we are also exploring and understanding the use of AI and machine learning for supervisory purposes, and therefore, we too need to understand the different forms of explainability tools that are available and their implications.”
Following her statement, the Federal Reserve Board along with four other financial regulatory agencies announced a request for information [viii]from financial institutions on their development, use, and governance of AI and machine learning.
[i] What is Machine Learning? by IBM Cloud Education, July 2020
[ii] Derisking machine learning and artificial intelligence, February 2019
[iii] Institute of the Future by Dell Technology, August 2019
[iv] https://www.mufg.jp/dam/ir/presentation/2018/pdf/slides190219_en.pdf
[v] https://www.ifc.org/wps/wcm/connect/e7e10e94-3cd8-4f4c-b6f8-1e14ea9eff80/45464_IFC_AML_Report.pdf?MOD=AJPERES&CVID=mKKNshy
[vi] https://www.ibm.com/downloads/cas/WKLQKD3W
[vii] https://www.federalreserve.gov/newsevents/speech/brainard20210112a.htm
[viii] https://www.federalreserve.gov/newsevents/pressreleases/bcreg20210329a.htm
As Product Marketing Specialist, Adalla is responsible for driving both the strategic and tactical aspects of RMA’s product sales. Prior to RMA, Adalla worked as a reporter for global media services and publication groups such as PEI Media and the Financial Times Group. She started her career at Campbell Lutyens, a global private capital advisory group. As an avid learner and a curious adventurer, Adalla speaks Korean, English, and Spanish, and has traveled to 19 countries. She graduated from Incheon National University with a Bachelor of International Trade in Northeast Asia Economic Studies.