Why Your Algorithms May Be Trained With Biases
4/23/2021
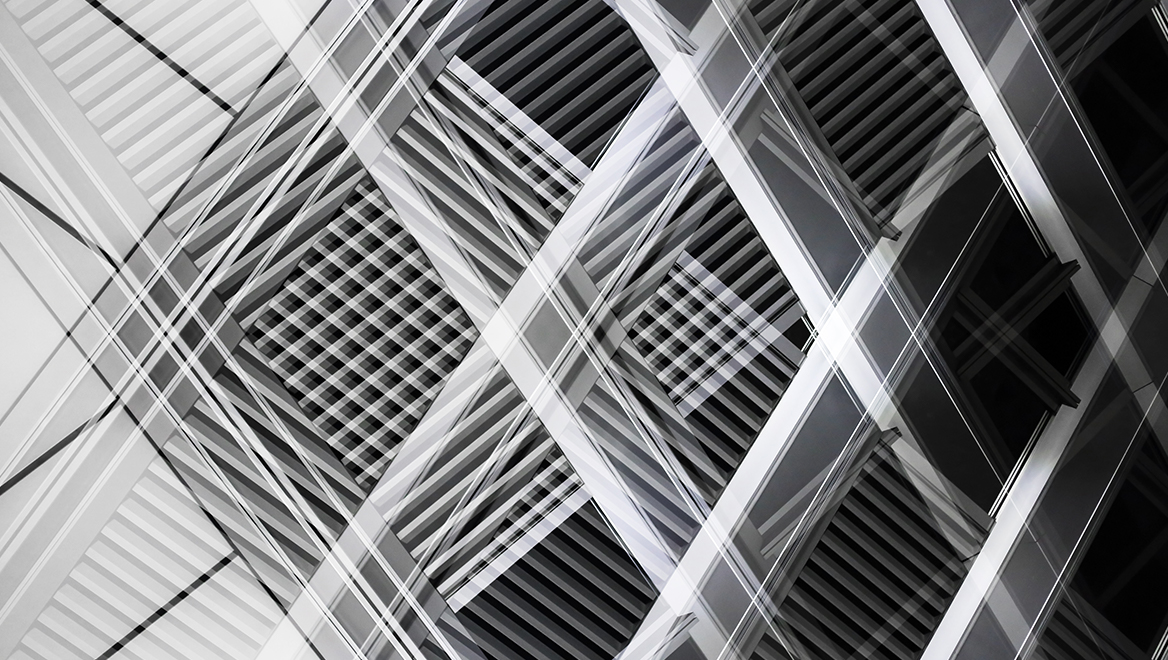
Here are the roles of bias, machine learning, and artificial intelligence that every lender should know.
In an ideal world, everyone would want their models to be fair and no one would want them to be biased, but what does that mean exactly? Unfortunately, there seems to be no universal and agreed upon definition of bias or fairness.
In the following interview between Fran Garritt of RMA and Kevin Oden of Kevin D. Oden & Associates, Garritt and Oden discuss the challenges of model risk management with a focus on consumer credit and machine learning.
Garritt: First, can you define bias in machine learning?
Oden: The best way to define bias in machine learning is really to think about how unfairness occurs in all modelling frameworks, machine learning just being one of them, and it is really bias or unfairness as an outcome of the model. So, when you think about fair – not showing favoritism towards an individual or group based on their intrinsic or acquired traits in the context of decision making is what fair means – and unfair is anything else. When we think about the models that we employ; they may have unfair outcomes.
Detecting that and correcting for it is one of the major tasks today as we use models more and more. Formulating fairness quantitatively in a model setting and a machine learning setting is difficult. But typically, one should start with the laws that are out there because there are laws out there which every bank has to adhere to.
Garritt: What do you consider are the contributing factors for bias in these models?
Typically, these models are trained on data and this data has been around in some cases for years and in some cases for decades in making these decisions. Unfortunately, people consciously or subconsciously are prone to bias in their decision-making process. So, it shows up in the data.
As an example, if a credit decision is made by an individual and that individual tends to give credit to people that they know or they like or they feel familiar with, then that becomes the training data for the credit decisioning models on a go forward basis.
When it is automated, you basically have automated prejudice in that model or bias in that model.
Garritt: I'm glad you brought up data and I'd like to explore that a little bit further. What is the importance of data and the future of AI machine learning and bias?
Oden: I just described how data that has been developed by humans can incorporate bias in it, and that data is currently being used in many automated decision-making processes. However, with machine learning and AI, people are going off and looking at it and incorporating it with, in many cases, great intentions to increase the credit space and increase the individuals who can have access to credit: the unbanked, if you will.
They are looking for a wide-ranging set of new data. We are all familiar with FICO scores. At least the credit folks are familiar with debt-to-income, loan to value, and late payments. But the machine learning folks and AI folks are looking for novel data and that increases the dataset. That can be good because it potentially brings new information which may give credit to those who are typically under banked and who are still very creditworthy.
However, that data may also increase bias if it is not monitored, if it's not utilized well, because a lot of the data that you see, for instance in social media, has the potential for digital redlining, and that is categorizing an individual in a particular protected class such as sex or race.
The increase in data is very important and it has many good outcomes if it is used appropriately and the analyst who makes these models understands the potential impacts and is able to correct for the potential downsides.
So, data is really important. Obviously, without data, none of this can work.
Garritt: With the perceived lack of regulatory guidance, what are some best practices that firms can follow regarding bias?
Oden: I have heard many people say that there does not seem to be a lot of regulatory guidance out there, but in fact, there really is. In the outcomes portion of it, you can think about the Equal Employment Opportunities Act, you can think about the Equal Credit Opportunity Act, the Fair Lending Act and on and on. Those acts, those in that legislation, basically determines in many respects what an equitable outcome is. So, the modeler has to take on the responsibility of demonstrating that the outcomes of their model adhere to those laws. And there are a number of ways to do that.
However, that really falls on the responsibility of the model and the model owner, the person who's going to utilize it to ensure that the outcomes are in line with those legislative acts and to help the model developer to do that.
There is the SR 11-7 (the Supervisory Guidance on Model Risk Management’s announcement in 2011) and the OCC 2011-12 (the Office of the Comptroller of the Currency’s guideline), which give a strong model risk management framework for how one should build a model, for how one should develop the model, and how one should validate that model. Again, bearing in mind that the outcomes of the model have to be in line with all of those acts that I just talked about.
I can guarantee you, when an examiner comes in from a fair lending perspective, whether it's the FDIC (the Federal Deposit Insurance Corporation) or the CFPB (the Consumer Financial Protection Bureau), they're going to make sure that there's no disparate impact, there's no disparate treatment, and that there isn't inappropriate steering that goes along with that credit decisioning process. That is something that the developer, validator, and the owner should all be concerned with and should be a part of the model development model and validation process.
Garritt: At RMA, we hear a lot about bias and the consumer credit space, and this [the consumer lending] is a space within banking where AI and machine learning is used quite extensively. So, what areas of consumer credit are most prone to bias?
Oden: Unfortunately, it is just about every area of consumer credit. But there are areas where you have an increased number of transactions, like consumer credit cards, where it can accelerate very quickly. So, the dollar in impact, the number of problems that occur in retail credit can increase very quickly.
The dollar impact for home equity and home mortgage bias is obviously extremely impactful as well from the dollar perspective on individuals. So, really all areas of consumer lending are very important. Even small business and small business lending can be easily impacted by unfairness and bias in the credit decisioning process. There is a lot less automation in those areas.
Fran Garritt is Director of Credit Global Markets Risk and Securities Lending at the Risk Management Association.
Kevin Oden is Founder and Managing Partner of Kevin D. Oden & Associates and Managing Director of RMA’s Model Validation Consortium.
STAY TUNED
At RMA’s Global Consumer and Small Business Risk Virtual Conference on May 5, 2021, this topic will be discussed further in a session, Use of Advanced Analytics, Machine Learning, and AI in Consumer Credit Decisioning. Stay tuned for upcoming RMA podcast episodes.
As Product Marketing Specialist, Adalla is responsible for driving both the strategic and tactical aspects of RMA’s product sales. Prior to RMA, Adalla worked as a reporter for global media services and publication groups such as PEI Media and the Financial Times Group. She started her career at Campbell Lutyens, a global private capital advisory group. As an avid learner and a curious adventurer, Adalla speaks Korean, English, and Spanish, and has traveled to 19 countries. She graduated from Incheon National University with a Bachelor of International Trade in Northeast Asia Economic Studies.